Maximizing efficiency with ai data quality audits in accounting: exploring how ai-driven data quality audits improve accuracy and save time
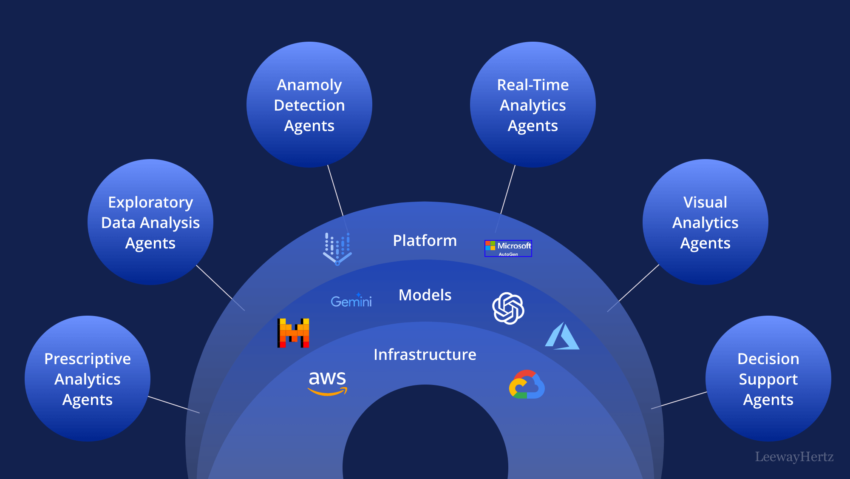
In the accounting industry, data quality is paramount. Inaccurate or incomplete data can lead to significant errors, regulatory non-compliance, and financial losses. Traditional methods of ensuring data quality are often time-consuming and prone to human error. However, advancements in artificial intelligence (ai) are transforming the landscape of data quality audits. Ai-driven data quality audits can significantly improve accuracy and efficiency, saving both time and resources. This blog explores how these ai-driven audits work and their benefits in the accounting field.
The importance of data quality in accounting
High-quality data is critical for accurate financial reporting, compliance with regulations, and effective decision-making. Poor data quality can result in:
Financial discrepancies: errors in financial statements can lead to misinformed decisions and financial losses.
Regulatory issues: non-compliance with regulatory standards due to inaccurate data can result in legal penalties.
Inefficiencies: time and resources spent on correcting data errors could be better utilized in strategic activities.
Traditional data quality audits: challenges
Traditional data quality audits in accounting involve manual processes that are often labor-intensive and error-prone. Common challenges include:
Time-consuming processes: manual audits require significant time and effort to review large volumes of data.
Human error: the likelihood of human error increases with the volume and complexity of the data.
Resource intensive: traditional audits often require substantial manpower, diverting resources from other critical tasks.
How ai-driven data quality audits work
Ai-driven data quality audits leverage machine learning algorithms and data analytics to automate the audit process. Here’s how they work:
1. Data collection and integration
Ai systems can automatically collect and integrate data from various sources, including accounting software, spreadsheets, and databases. This integration ensures that all relevant data is considered in the audit process.
Example: an accounting firm uses ai to collect financial data from multiple erp systems, ensuring a comprehensive audit scope.
2. Data cleaning and preparation
Ai tools can clean and prepare data by identifying and correcting errors, such as duplicates, missing values, and inconsistencies. This step ensures that the data set is accurate and reliable.
Example: ai algorithms detect and correct inconsistencies in financial records, such as mismatched entries and incomplete transaction details.
Statistical insight: according to a report by gartner, automated data preparation can reduce data errors by up to 40%.
3. Data analysis and anomaly detection
Ai-driven audits use machine learning models to analyze data and identify anomalies or patterns that may indicate errors or fraudulent activities. These models can continuously learn and improve over time, enhancing their accuracy and efficiency.
Example: ai identifies unusual transaction patterns that deviate from historical data, flagging them for further review by auditors.
Statistical insight: a study by deloitte found that ai-driven anomaly detection can improve the accuracy of fraud detection by 30%.
4. Real-time monitoring and reporting
Ai systems provide real-time monitoring and reporting capabilities, allowing accountants to track data quality continuously. Automated reports highlight areas that require attention and provide actionable insights.
Example: an ai-powered dashboard provides real-time updates on data quality metrics, alerting accountants to potential issues as they arise.
Statistical insight: according to mckinsey, real-time monitoring can reduce the time spent on data quality management by 50%.
Benefits of ai-driven data quality audits
1. Improved accuracy
Ai-driven audits significantly reduce the likelihood of human error, ensuring more accurate and reliable data. This accuracy is crucial for financial reporting and compliance.
Example: an accounting firm experiences fewer errors in financial statements due to the precision of ai-driven audits.
2. Time savings
Automation of data quality audits saves considerable time, allowing accountants to focus on higher-value tasks, such as analysis and strategic planning.
Example: ai reduces the time spent on routine data checks, freeing up auditors to concentrate on complex financial analysis.
Statistical insight: according to a report by pwc, automation in auditing can reduce audit time by up to 70%.
3. Cost efficiency
By reducing the time and resources required for data quality audits, ai-driven solutions can lead to significant cost savings for accounting firms.
Example: a mid-sized accounting firm reduces its audit-related expenses by implementing ai-driven data quality tools.
Statistical insight: a survey by accenture found that companies using ai in financial processes saw a 20% reduction in operational costs.
4. Enhanced compliance
Ai-driven audits ensure that data quality standards are consistently met, helping firms maintain compliance with regulatory requirements and avoid legal penalties.
Example: ai ensures that all financial transactions comply with relevant accounting standards and regulations, reducing the risk of non-compliance.
Implementing ai-driven data quality audits
1. Selecting the right ai tools
Choosing the appropriate ai tools is crucial for successful implementation. Consider factors such as ease of integration, scalability, and the specific features offered by different tools.
Key points:
Integration: ensure the ai tool can seamlessly integrate with existing accounting systems.
Scalability: choose a solution that can scale with the growth of your business.
Features: look for tools that offer comprehensive data quality management capabilities, including cleaning, monitoring, and reporting.
Example: an accounting firm selects an ai-driven audit tool that integrates with their erp system and offers real-time monitoring and anomaly detection.
2. Training and support
Provide comprehensive training to ensure that your team can effectively use ai tools. Ongoing support is also essential to address any challenges that arise.
Key points:
Initial training: offer training sessions to familiarize staff with the new ai tools and their functionalities.
Ongoing support: provide continuous support to help staff navigate and utilize the ai tools effectively.
Example: a firm conducts training workshops for auditors, followed by regular q&a sessions to address any issues.
3. Continuous monitoring and improvement
Regularly review and optimize the use of ai tools to ensure they continue to meet your data quality needs.
Key points:
Performance monitoring: continuously monitor the performance of ai tools and make adjustments as needed.
Feedback loop: establish a feedback loop where auditors can provide input on the ai tools and suggest improvements.
Example: a firm regularly reviews ai-driven audit reports and gathers feedback from auditors to refine and improve the tools.
Conclusion
Ai-driven data quality audits offer significant advantages for the accounting industry, including improved accuracy, time savings, cost efficiency, and enhanced compliance. By automating routine tasks and providing real-time insights, ai tools enable accountants to focus on higher-value activities and make informed decisions. Implementing the right ai tools, providing comprehensive training, and continuously monitoring and optimizing their use are essential steps to maximizing the benefits of ai-driven data quality audits.